AI in Reinsurance 2024
AI in Reinsurance 2024
Emerging technologies often generate significant buzz, but their real-world impact can lag behind the hype. GenAI is no exception.
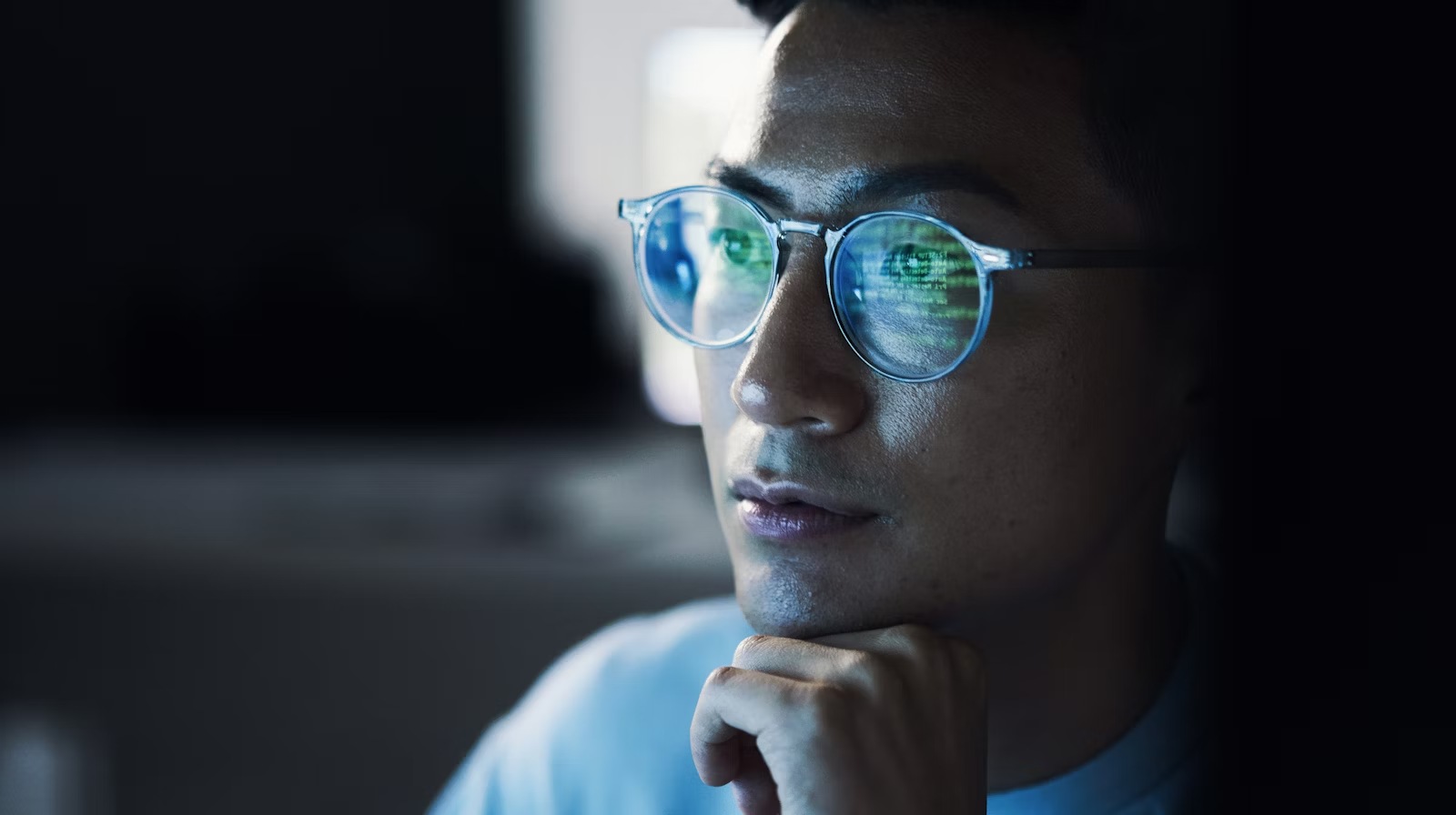
In a recent fireside chat, Jensen Huang, CEO of Nvidia, and Mark Zuckerberg, CEO of Meta, eagerly agreed with one another that in future every business will have an AI (RD, 2024). Whilst they may have a couple of llamas in the race, analysis from leading financial organizations does also support this. The impact of the AI revolution is estimated to raise global GDP by 7%-10% over a 10-year period and regulating it has become a priority for governments around the world (GS, 2023) (JPM, 2024).
Yet for the companies deploying GenAI solutions, getting there seems to be taking longer than anticipated (WSJ, 2024). A report by Gartner published in June described the current stage of the GenAI hype as the “trough of disillusionment” (Gar, 2024) – a stage of the hype cycle characterised by peak hype but with presently limited impact. The upshot of this is that there is a lot of work to be done.
This is not news for re/insurance carriers, brokers and insurtechs. An EY Parthenon study published in May found that 99% are already investing in, or planning to invest in GenAI, with more than 70% allocating at least $5m (EY, 2024). Combined with strategic partnerships such as that between AXA and GenAI startup Mistral (PCA, 2024), the re/insurance industry is demonstrating a serious and long-term commitment to building with these technologies.
Nature of change for reinsurance
With the release of ChatGPT nearly two years ago, advancements in GenAI were quickly recognised as laying the foundations for transforming how unstructured data is processed in reinsurance. Since then, Indico Data have launched a monthly benchmarking exercise that highlights the performance of Large Language Models (LLMs) in unstructured data processing tasks (Ind, 2024). These tasks, ranging from data extraction to classification and summarization, demonstrate the ability of GenAI to transform processes involving unstructured data.
This year, as a growing number of AI applications in re/insurance are conceived and implemented, this article explores three themes:
- Picking the right tasks;
- Developing the ability to build with AI; and
- Collaboration.
Picking the right tasks
Research by Oxbow Partners in July found that carriers do not feel prepared to execute on the transformational opportunity that GenAI presents. While 86% of carriers expect GenAI to be transformative over a 5+ year time horizon, only 14% of specialty and reinsurance carriers are in a place where target use cases are clearly defined and buy-in from the business is secured (OxP, 2024).
To help define target use cases in the US Government, a team from Deloitte proposed a framework for identifying tasks amenable to GenAI from the publicly available O*Net database. Within this framework, tasks were scored across three measures: creative difficulty, context variability, and the need for accuracy. Tasks with moderately high creative difficulty, moderate context variability and moderate accuracy were classified as potentially good candidates for GenAI (Del, 2024).
Adopting a variation of this framework, analysis at Price Forbes Re has identified over 5,000 tasks common across the reinsurance industry with potential to be a good fit for augmentation with existing GenAI technologies. This assessment is based on a mixture of expert human labelling, proprietary machine learning algorithms and additional scoring metrics that capture the interpersonal nature of many reinsurance tasks.
As well as tasks such as reviewing contracts and underwriting reports, this adapted framework highlights less obvious target use cases like writing SQL scripts to query servers of exposure databases. Retrieval Augmented Generation (RAG) supported chatbots are a relatively common application of GenAI that use search capabilities to enhance the relevance and accuracy of LLM generated responses. This analysis highlights how code generation technologies could extend the functionality of these applications from search to query, for example enabling real-time requests such as “return me a table of all locations from exposure database A that exist in both portfolio B and portfolio C”. The application of GenAI to such tasks that would traditionally have required coding skills could significantly reduce the friction to basic information for those in positions such as reinsurance underwriting.
This is just one of many new and powerful capabilities that can be integrated into common workflows. As another example, consider the following sequence of tasks that a catastrophe modeller at a broker might be required to perform:
- Design an appropriate and tailored modelling protocol with the client,
- Clean, preprocess, transform and validate exposure data received from the client,
- Import the data into catastrophe modelling software such as RMS or AIR,
- Adjust model parameters based on the initial simulation results and validation feedback, and
- Present results back to the client.
Each of these tasks score differently on creative difficulty, context variability and need for accuracy.
How these tasks score along each measure provides a framework for determining the potential for the task to be augmented with GenAI or other technologies. This is not definitive but can provide a useful guide.
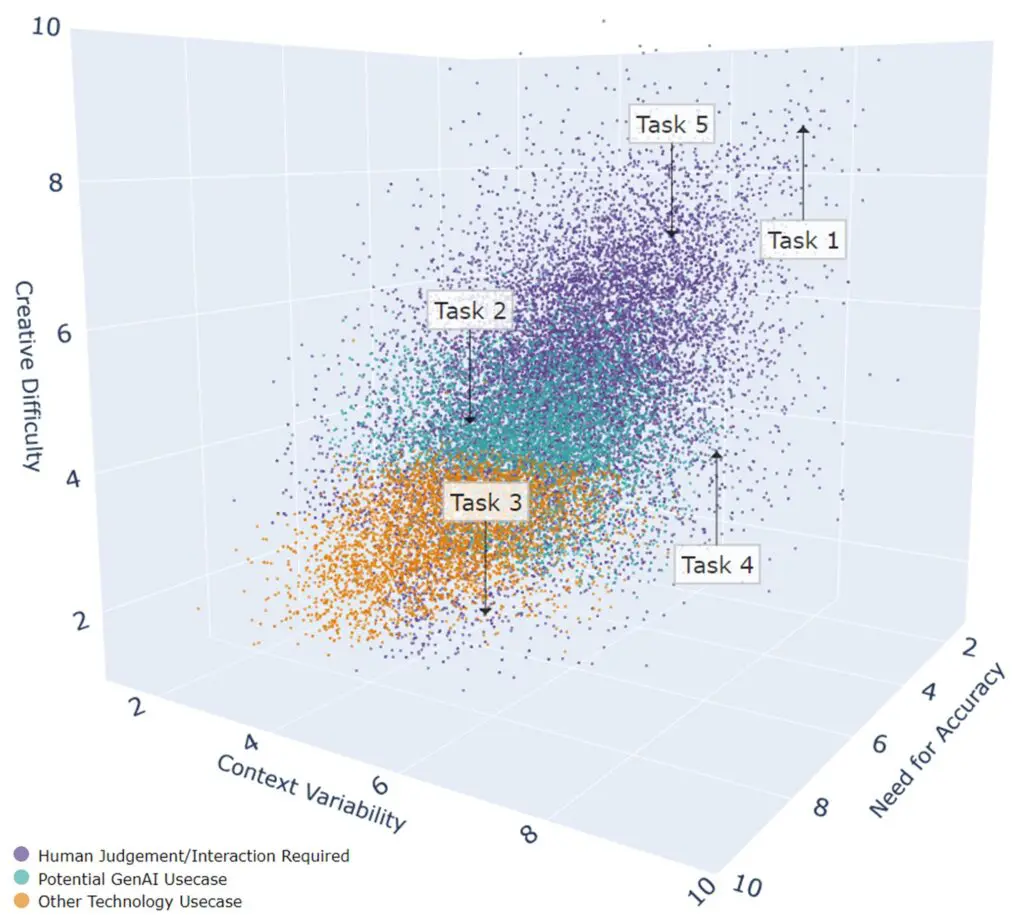
For example, task 1 scores highly on creative difficulty which means a significant degree of human judgement will still be required, while task 2 scores more moderately across all measures which makes it a good potential candidate for augmentation with GenAI. Task 3 scores low on creative difficulty and context variability but highly on the need for accuracy, which makes it a good candidate for augmentation with other technologies like robotics, but not GenAI.
Most, if not all jobs and processes in reinsurance can be described by a similar sequence of diverse tasks with varying degrees of context variability, creative difficulty and need for accuracy.
Developing the ability to build with it
This extension of the original analysis by Deloitte demonstrates why GenAI will increasingly augment most job roles in reinsurance.
With existing technology, multiple GenAI models are generally needed to perform a diverse range of specialized tasks to a satisfactory standard. Therefore, the ability to be able to build custom solutions is key. As the power of GenAI increases, and the cost of using it decreases, the ability to ensure these custom solutions scale with, and benefit from inevitable technological advancements will be essential. The re/insurance industry, as already being spearheaded by some of the larger players and key innovators, needs to remain adaptable.
One approach to adapting in the short term is to purchase specialist vendor solutions. These solutions may provide quick wins for innovation in some cases, but risk being outpaced by foundational advances and economically inefficient for adapting to AI in the longer term.
Initiatives developed at Price Forbes Re suggest that using GenAI, in-house solutions can often achieve most of, and in some cases exceed, the capabilities of existing vendor solutions at a fraction of the cost. In the buy-vs-build debate, these results suggest that the balance may tilt more towards building in-house AI solutions. This has the potential to not only reduce costs but also upskill in-house developers to more quickly adopt changes in technology and customise solutions to specific needs. Particularly as the abilities of foundation models outpace vendor solutions.
Collaboration
The ability to build in-house solutions will require an influx of new talent and expertise into reinsurance. The existing talent gap is already a well-established concern, with 50% of the current workforce estimated to retire over the next 15 years (InJo, 2024). So, it’s perhaps unsurprising that talent and expertise in AI were also revealed as the biggest concern for carriers building the foundations for GenAI, with an average readiness score of just 4.4 out of 10 (OxP, 2024).
Despite the scarcity of talent and expertise, AI teams are anecdotally duplicating efforts and building copies of the same baseline solutions to the same problems. Instead of duplicating efforts, collaboration in common areas of interest has been suggested by some industry leaders as a more effective way for carriers to enter the fast-moving world of AI (CaM, 2024).
Whilst a carrier’s competitive advantage is based on their ability to profitably underwrite risk, rather than to build AI tooling, it is becoming necessary to have both sets of skills. This is also true for brokers and insurtechs who will need to be adaptable to advancements in AI to remain competitive at the core of their business.
For those doing both already, substantial AI innovation over the next 5+ years seems likely to create meaningful value. For those in the so-called “trough of disillusionment”, collaborating on common foundational solutions could be a helpful way forward.
Conclusion
While the transformative potential of AI has yet to be fully realised, the re/insurance industry is laying the groundwork for future advancements. Significant investments and strategic partnerships are driving progress, but as firms navigate through the “trough of disillusionment”, selecting the right tasks, developing the ability to build with GenAI and fostering collaboration will be pivotal. The work continues.
Written by Oliver Mapp and Mahboob Mian.
A strategic broking partner
Price Forbes Re uses both local open-source and closed-source private and secure instances of the leading GenAI foundation models to facilitate partnerships with clients on a range of AI and data science services.
AI use cases that have a high need for accuracy, such as risk assessment, claims management and automated underwriting, inherently carry a higher burden of risk due to the critical nature of these processes. To help clients guarantee the performance of AI solutions we have partnered with Munich Re on the sale of their Insure AI product and have market-leading expertise in Cyber risk.
To discuss collaboration in areas of common interest.
To discuss the impact of AI on Cyber risk.
tom.johansmeyer@priceforbesre.com
To discuss options for guaranteeing performance of AI solutions.
References
CaM. 2024. [Online] 4 March 2024. [Cited: 1 8 2024.] https://www.carriermanagement.com/features/2024/03/04/258492.htm.
Del. 2024. [Online] 25 April 2024. [Cited: 1 8 2024.] https://www2.deloitte.com/us/en/insights/industry/public-sector/automation-and-generative-ai-in-government.html#generative-ai-and-government-work.
EY. 2024. [Online] 14 May 2024. [Cited: 1 8 2024.] https://www.ey.com/en_us/insights/insurance/how-to-revolutionize-the-insurance-value-chain-with-generative-ai.
Gar. 2024. [Online] 17 June 2024. [Cited: 1 8 2024.] https://www.gartner. com/en/documents/5505695.
GS. 2023. [Online] 5 April 2023. [Cited: 1 8 2024.] https://www.goldmansachs.com/insights/articles/generative-ai-could-raise-global-gdp-by-7-percent.html.
Ind. 2024. [Online] 2024. [Cited: 1 8 2024.]
InJo. 2024. [Online] 4 March 2024. [Cited: 1 8 2024.] https://www.insurancejournal.com/magazines/mag-features/2024/03/04/762494.htm.
JPM. 2024. [Online] 14 February 2024. [Cited: 1 8 2024.] https://www.jpmorgan.com/insights/global-research/artificial-intelligence/generative-ai.
OxP. 2024. [Online] July 2024. [Cited: 1 8 2024.]
PCA. 2024. [Online] 25 June 2024. [Cited: 1 8 2024.]
RD. 2024. [Online] 30 July 2024. [Cited: 1 8 2024.]
WSJ. 2024. [Online] 25 June 2024. [Cited: 1 8 2024.]